Data Science Principles
Are you prepared for our data-driven world?
Data Science Principles is a Harvard Online course that gives you an overview of data science with a code- and math-free introduction to prediction, causality, data wrangling, privacy, and ethics.
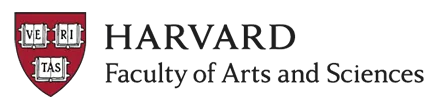
4–5 hours per week
4–5 hours per week
What You'll Learn
What is data science, and how can it help you make sense of the infinite data, metrics, and tools that are available today?
Data science is at the core of any growing modern business, from health care to government to advertising and more. Insights gathered from data science collection and analysis practices have the potential to increase quality, effectiveness, and efficiency of work output in professional and personal situations.
Data Science Principles makes the foundational topics in data science approachable and relevant by using real-world examples that prompt you to think critically about applying these understandings to your workplace. Get an overview of data science with a nearly code- and math-free introduction to prediction, causality, visualization, data wrangling, privacy, and ethics.
Data Science Principles is an introduction to data science course for anyone who wants to positively impact outcomes and understand insights from their company’s data collection and analysis efforts. This online certificate course will prepare you to speak the language of data science and contribute to data-oriented discussions within your company and daily life. This is a course for beginners and managers to better understand what data science is and how to work with data scientists.
Data Science Principles is part of our Harvard on Digital Learning Path.
The Harvard on Digital course series provides the frameworks and methodologies to turn data into insight, technologies into strategy, and opportunities into value and responsibility to lead with data-driven decision making.
The course is part of the Harvard on Digital Learning Path and will be delivered via HBS Online’s course platform. Learners will be immersed in real-world examples from experts at industry-leading organizations. By the end of the course, participants will be able to:
- Understand the modern data science landscape and technical terminology for a data-driven world
- Recognize major concepts and tools in the field of data science and determine where they can be appropriately applied
- Appreciate the importance of curating, organizing, and wrangling data
- Explain uncertainty, causality, and data quality—and the ways they relate to each other
- Predict the consequences of data use and misuse and know when more data may be needed or when to change approaches
Your Instructor
Dustin Tingley is a data scientist at Harvard University. He is the Thomas D. Cabot Professor of Public Policy with a joint appointment in the Harvard Kennedy School of Public Policy and Harvard Government Department. Professor Tingley is Deputy Vice Provost for Advances in Learning and helps to direct Harvard's education focused data science and technology team. He has helped a variety of organizations use the tools of data science and helped to develop machine learning algorithms and accompanying software for the social sciences. He has written on a variety of topics using data science techniques, including education, politics, and economics.
Real World Case Studies
Affiliations are listed for identification purposes only.
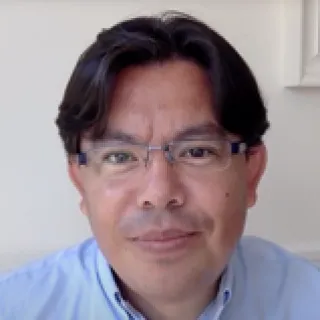
Mauricio Santillana
Listen to Harvard Professor and faculty member at Boston Children’s Hospital analyze Google Flu, its failures, and lessons learned.
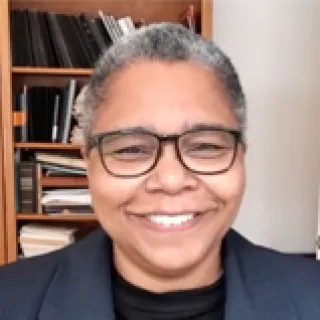
Latanya Sweeney
Explore the difficulties faced in keeping data anonymous and private with Harvard Professor and Director of the Data Privacy Lab in IQSS at Harvard.
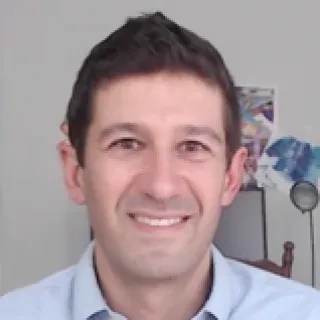
Dan Restuccia
Learn how Burning Glass Technologies uses text analysis to recommend job openings, skill development, and labor market trends.
Available Discounts and Benefits for Groups and Individuals
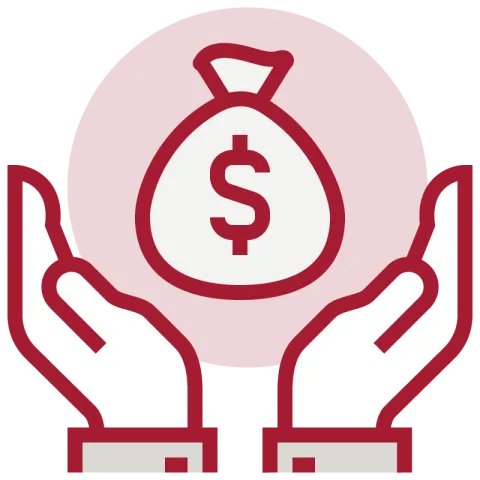
Experience Harvard Online by utilizing our wide variety of discount programs for individuals and groups.
Past Participant Discounts
Learners who have enrolled in at least one qualifying Harvard Online program hosted on the HBS Online platform are eligible to receive a 30% discount on this course, regardless of completion or certificate status in the first purchased program. Past Participant Discounts are automatically applied to the Program Fee upon time of payment. Learn more here.
Learners who have earned a verified certificate for a HarvardX course hosted on the edX platform are eligible to receive a 30% discount on this course using a discount code. Discounts are not available after you've submitted payment, so if you think you are eligible for a discount on a registration, please check your email for a code or contact us.
Nonprofit, Government, Military, and Education Discounts
For this course we offer a 30% discount for learners who work in the nonprofit, government, military, or education fields.
Eligibility is determined by a prospective learner’s email address, ending in .org, .gov, .mil, or .edu. Interested learners can apply below for the discount and, if eligible, will receive a promo code to enter when completing payment information to enroll in a Harvard Online program. Click here to apply for these discounts.
Gather your team to experience Data Science Principles and other Harvard Online courses to enjoy the benefits of learning together:
- Single invoicing for groups of 10 or more
- Tiered discounts and pricing available with up to 50% off
- Growth reports on your team's progress
- Flexible course and partnership plans
Syllabus
Data Science Principles makes the fundamental topics in data science approachable and relevant by using real-world examples and prompts learners to think critically about applying these new understandings to their own workplace. Get an overview of data science with a nearly code- and math-free introduction to prediction, causality, visualization, data wrangling, privacy, and ethics.
- Study a flu detection case study alongside Professor Dustin Tingley and Mauricio Santillana, Assistant Professor at Harvard’s T.H. Chan School of Public Health.
- Explain why data collection is important.
- Identify factors that may affect data quality.
- Recognize that not all data is numerical.
- Explain how the organization of data can affect the information you are able to extract from it.
- Study a predicting sepsis case alongside Craig Umscheid, Vice President and Chief Quality and Innovation Office, University of Chicago Medicine.
- Understand the basic structure of a predictive algorithm.
- Identify where human decisions shape predictive systems.
- Evaluate the success of a predictive system.
- Study The Google Tax Case.
- Explain why it is important to establish causal relationships.
- Identify barriers to establishing causal relationships in a variety of settings.
- Identify why randomization can help establish a causal relationship but also create other problems.
- Explore a privacy and facial recognition case study with Latanya Sweeney, Professor of the Practice of Government and Technology at the Harvard Kennedy School and Sciences, director and founder of the Public Interest Tech Lab, and director and founder of the Data Privacy Lab.
- Explain why data privacy is important.
- Describe what can constitute a violation of privacy.
- Critique existing privacy policies.
- Create a set of ethical tenets to guide data work at their own organizations.
- Study the Burning Glass and Text Data case.
- Identify sources of non-numerical data.
- Explain why it would be useful to use non-numerical data.
- Describe the differences in approach for supervised and unsupervised learning.
- Identify use cases for neural networks.
- Explore a case study on reducing food waste with Shelf Engine.
- Describe some algorithms commonly used in data science.
- Understand basic workhorse algorithms in data science such as regression.
- Explain why and how such tools are made substantially more complex.
- Explain the crucial role humans have in overseeing and maintaining algorithms.
- Explain some of the trade-offs between more sophisticated algorithms, including the costs of running and evaluating their success.
- Learn about the Harvard Link case study.
- Explain the importance of data transformation and wrangling.
- List the common technologies used within data science ecosystems.
- Describe the connection between data science tasks, software tools, and hardware tools.
- Identify potential sources of bottlenecks in the data science process.
- Work on a health care prioritization case study.
- Recognize a problem that an algorithm might be able to solve.
- Recognize the challenges created by using data science tools in ways outside their intended use.
- Identify steps within the data science process that need auditing.